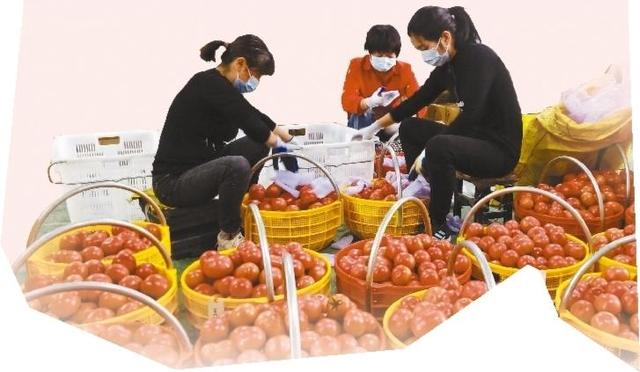
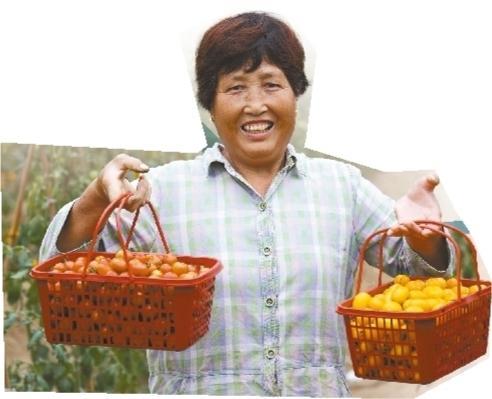
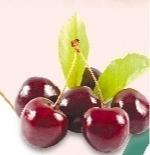
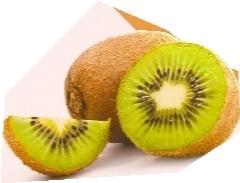
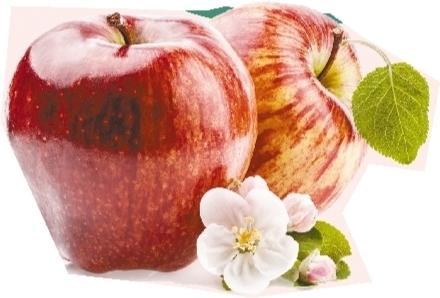

There are more and more green cars on the road.
Take Shanghai as an example. In 2023, the number of new energy vehicles in the city reached 1.288 million, ranking first in the world. In 2016, this number just exceeded 100,000, an increase of nearly 12 times in seven years.
Counting the history, it has been ten years since the first round of explosive growth of new energy vehicles in 2014.
In 2014, stimulated by the intensive subsidy policies of the central government and local governments, the demand for new energy vehicles was greatly released, which ushered in both production and sales. According to the statistics of China Automobile Association, in 2014, 78,499 new energy vehicles were produced and 74,763 vehicles were sold, up 3.5 times and 3.2 times respectively over the previous year.
The development of new energy vehicles has also brought economic benefits to their cities.In the past ten years, countless cities have come to an end. More than 10 cities, including Shanghai, Chongqing, Shenzhen and Xi ‘an, have set the goal of building a "new energy automobile capital", hoping to get this big cake first.
So in the past ten years, which cities have successfully embarked on their own development path of new energy vehicles? Based on corporate big data, we sketched out a ten-year urban competition record.
Ten Years of Enterprise Big Data: Counting Four Major Urban Industrial Clusters
To build a new energy automobile industry, one of the most important goals is to form industrial agglomeration, so as to achieve scale effect. We selected four major urban agglomerations with the largest concentration in China’s new energy vehicle industry, and further analyzed 62 cities in the Yangtze River Delta region, Pearl River Delta region, Beijing-Tianjin-Hebei region and Chengdu-Chongqing region.
According to the industrial database of CBN New First-tier City Research Institute, from the latest data of new enterprises such as "high-tech enterprises", "small and medium-sized scientific enterprises" and "specialized and special new enterprises",By 2022, there were 5,764 new enterprises related to new energy vehicles in the four industrial zones.
Based on the industry classification algorithm, the database matches enterprises to all segments of the new energy automobile industry chain, in which enterprises can be matched to three major industries according to the upstream, downstream and downstream: auto parts, vehicle manufacturing and service and application.
In order to better compare the different development directions of cities in the three major industries, we calculated the median of "total registered capital of new energy vehicle enterprises" in 62 cities in the three major industries, which were 947 million yuan, 297 million yuan and 735 million yuan respectively. When we calculate and compare the total registered capital of the three major industries in each city, we can see the industrial distribution characteristics of each city, thus calculating the main industries in the direction of new energy vehicles. For example, the total registered capital of new-type enterprises of "service and application" in Shanghai is 11.561 billion yuan, which is 16 times of the above median; However, the total registered capital of new enterprises related to "auto parts" and "vehicle manufacturing" in Shanghai is only 12 times and 13 times of the above median. Therefore, "service and application" can be considered as the main industry of new energy vehicles in Shanghai.
In order to compare cities better, we spread these data on the map. The main industries in each city are distinguished by different colors, and the total registered capital of emerging enterprises is represented by the size of a circle. It can be found that,The Yangtze River Delta urban agglomeration has more cities with new energy vehicles, and its geographical coverage is also wider.
In addition, among the 62 cities, 25 cities are mainly located in the downstream service and application industries, including Guangzhou, Shanghai, Shenzhen and Chengdu, with the largest number of cities. The main industries in 17 cities are the upstream auto parts industry, including Zhenjiang, Yangzhou and Taizhou. The main industries in 20 cities are the automobile manufacturing industry in the middle reaches, including Beijing, Tianjin and Changzhou.
Then if we add the time dimension, what about the changes of industrial clusters in four major cities from the span of ten years?
We calculated the annual growth rate of the number of new enterprises in industrial clusters in cities compared with 2013, and found that,"Automobile Manufacturing" and "Service and Application" located in the middle and lower reaches of the industrial chain have always been the development focus of the Yangtze River Delta region and Beijing-Tianjin-Hebei region in recent ten years. Comparatively speaking, the Pearl River Delta region has made greater efforts to develop the service and application industries, while Chengdu-Chongqing region has made greater efforts in the vehicle manufacturing industry.
The battle for "the capital of new energy vehicles": Who will end up? Who won?
In the battle for new energy automobile cities, cities have tried their best: some cities have made great efforts to develop with the help of their huge consumer market; Some cities actively promote industrial transformation and upgrading based on local industrial characteristics; Some cities tap the advantages of resources to attract investment.
So which cities won in this competition? Where did you win again? Judging from the available public data,Among the top cities of new energy vehicles, the top three are Xi ‘an, Changsha and Shenzhen, and the top three are Shenzhen, Liuzhou and Shanghai.
Capital never sleeps. In the face of new economic growth points full of infinite possibilities, in the past ten years, the new energy vehicle market has been surging, and many cities have also made efforts to compete for the "capital of new energy vehicles", and the industrial battle has continued to start. We look forward to the future new energy automobile industry, adhering to the concept of innovation and sustainability, and developing healthily and vigorously.
On January 11th, Rome Green Finance (ROMA) rose by 21.38% in intraday trading, reaching $3.52 per share at 00:11, with a turnover of $24,058,300.
According to financial data, as of September 30, 2022, Rome’s total green financial income was HK$ 6,200,600, a year-on-year decrease of 0.68%; The net profit returned to the mother was-HK$ 693,600, a year-on-year increase of 62.34%.
This article comes from: financial circles
Author: American stock king
Keeping up with the fashion trend and understanding the more fashionable clothing collocation methods will make you become fashionable slowly. There are actually many fashionable clothing styles, especially in Paris.The capital of fashion, dareThere are also many people who challenge their own clothes. Paris street amateurs wear and share, which is worthy of being the fashion capital, and "asexual" wearing is too fashionable.
It turns out that what is popular in Paris is "asexual" clothing.Each set is unique.Both men and women can wear it, and the matching way of asexual wear and neutral wind is similar, but it is more difficult to distinguish clothing.Sense of men and womenAt the same time, most of the neutral clothes are worn by women, but the asexual clothes are clothes styles that can be worn by both men and women.
Handsome and generous short cotton-padded jacket+sweater
The short cotton-padded jacket itself is a gender-neutral clothing style, and its design is very simple. The fluffy design also makes the temperament more energetic. Match upTrousersAfter that, the temperament is very handsome, but because it is matched with a sporty suit, the temperament is much softer, and with the high waist design, the effect of clothing collocation is certain.Elegant and feminine.
ZaipeiAsexualWhen wearing clothes, choose colorful scarves to increase the sense of handsomeness, and you can blur the clothes.Boundaries between men and women.
Fashion personality of western-style clothes
A suit is not only a suit of the same style for men and women, but also a handsome and cool atmosphere. As a menswear, it can be paired with black wide-leg pants, showing a generous and steady temperament. As a woman’s dress, it can be worn with a lady’s shirt., enlarge the elegant gas field.
The way of overlapping can make the suit easily show the asexual temperament, and the number of shirts, jackets and ties inside has increased.Casual natureThe effect. You can also make the gas field more by matching boots and ladies’ handbags.Soft and fashionable.
Leather jacket and jeans
The long coat version design is very simple, and the clean lines are also very good.Handsome,However, if the leather design is not a big design, the effect it can present will become a bit.womanTaste, through the collocation of very handsome pants, can effectively alleviate this temperament.
Fat dark jeans can not only show handsome atmosphere, but also retro literature and art, and the coat with leather design is very unique. Simply matching it with a black shirt or a T-shirt is enough.fashionPut on a yellow shirt and the visual effect will be more novel.
Asexual collocation of windbreaker
The fabric of trench coat will be thinner than that of coat, and the matching clothes will be more handsome, and the gas field will be very generous and masculine. At the same time, windbreaker is also very suitable for deviation.YujiefengThe collocation method, as long as the combination of men’s and women’s clothing, can be done.The characteristics of asexuality.
For example, if you choose tight pants or pipe pants, you can wear a trench coat with a certain femininity, and at the same time,It looks free and easy.
Sporty coat and jeans
When matching clothes with a handsome sporty style, you can wear a asexual style by choosing a neutral style with a simple design and a darker color, but remember to choose a fashionable style when matching pants, and you can also match one.Free and easy circumferenceTowel, or a bigger bag, to make the temperament look feminine.
When choosing a sporty coat, it is recommended to wear a baseball cap or a short coat with velvet, which can better show it.sluggishAt the same time, it is more advanced.
Black coat and men’s belt
The shoulder pad design of suits and coats can make the gas field more.seniorThe effect of matching the invisible wind is also very easy, but there is a small accessory that many people will ignore, that isA man’s beltThe design is relatively handsome and simple, and the temperament is even more unique after wearing a coat.
There is no problem in wearing the same style for men and women or a little feminine in other clothing collocation methods. The unique temperament is very attractive. The design of the belt is the crowning touch in clothing collocation, but remember to choose a coat with obvious silhouette or shoulder pad design.The matching gas field will be more unique.
A short vintage coat
The design of short and medium models will naturally show that it is betweenMasculinity and femininityBetween the gas field, the short design can also be matched with the neutral color of brown, and the temperament is more unique, andrestore ancient waysThe effect. However, remember to choose thick fabrics, and the style of clothing should be cut simply, so there can be noThe design of waist-closing.
When matching, it will be better to choose fat wide-leg pants that can cover up the figure curve, and the effect will be very natural.
Western-style clothes
Suits are very suitable for matching.SexyandasexualFeeling of clothing, with asexual clothing, the most taboo is the design of the clothing style.Too handsome,In fact, it is good to choose a more casual collocation method, which can also make the momentum more elegant, and the temperament that can be displayed is also very literary and casual.
Does your child like football? Does your child want to be a caddy with professional league experience? Do your children want to be caddies for Guangzhou team? If everything comes true, I believe your child will have an unforgettable memory, which will be an important, meaningful and valuable experience on his growth path. I am afraid it was difficult to achieve it in the past, but now the opportunity has come! Guangzhou Football Club, which has been more enthusiastic about public welfare since this year, will now give you such a ready-made opportunity.
—— On June 4th, 2023, at 15:00, Guangzhou Yuexiu Stadium, the first-class league of Chinese Football Association, Guangzhou Team vs Jinan Xingzhou Guangzhou Langqi & Guangzhou Football Club players entered the arena and held hands to select caddies. The limited registration channel has been opened. Fans are welcome to let their children participate. Please see:Guangzhou team started to sign up for caddy selection activities hand in hand!(Click below to read the original text to view)
Welcome everyone to sign up and let their children have a unique new caddy experience!
With the opening of [Video Number], we will share all the interesting and meaningful moments around us in the future, including Guangzhou Team, Hongyuan and Jielun … Sports, entertainment, film and television entertainment are all available, and everyone is welcome to support and pay attention.
Some people watch the game and only watch the "big score".
In the WTT Macau Championship in 2023, Sun Yingsha lost 4-0 to Chen Meng in the women’s singles semi-final, and some people felt abnormal.
Last week’s WTT Xinxiang Championship was also a semi-final in women’s singles. Chen Meng lost 4-0 to Sun Yingsha. I wonder if they also felt abnormal?
In WTT Xinxiang Championship, Sun Yingsha scored 4-0 in Chen Meng, and the scores of the four sets were 11-5, 11-5, 11-7 and 11-6.
The score of four games failed to hit the key points, so it can be said that Chen Meng was completely defeated.
In the WTT Macau Championship, Sun Yingsha of Chen Meng scored 4-0, and the scores in four sets were 16-14, 11-6, 11-8 and 11-9.
Chen Meng and Sun Yingsha won the first game 10-9, and then Chen Meng tied the game and won five games in a row, only to win narrowly 16-14.
Sometimes, a small score per game is more telling.
Compare another women’s singles semi-final, although Manyu Wang won Wang Yidi 4-2, but this game is less suspense than Chen Meng’s 4-0 win over Sun Yingsha.
Manyu Wang 4-2 Wang Yidi, the score of six games: 7-11, 11-9, 11-9, 11-6, 5-11, 11-4.
Manyu Wang is tall and arm-length, and her movements will not be restored very quickly, which determines that her forehand and backhand cohesion will not be very smooth in the near stage. What Manyu Wang is afraid of is the players with fast convergence speed and not weak strength. In the face of fast players, she often has to quit the stage and exchange distance for reaction time.
Only with speed and strength, it is possible to penetrate Manyu Wang’s defense.
However, Wang Yidi is not a speed player, and her playing style also depends on the quality of her own veneer, which is similar to that of Manyu Wang.
However, compared with Wang Yidi, Manyu Wang has stronger rotation in returning the ball and higher veneer quality. From a fundamental point of view, Manyu Wang’s strength is higher than Wang Yidi’s.
Therefore, regardless of Manyu Wang’s four-to-several win, as long as it is a best-of-seven game, the chance of surprise is very small. Under normal circumstances, it is only a matter of time before Manyu Wang defeats Wang Yidi.
However, in the semi-final between Chen Meng and Sun Yingsha, after 9-9 in the fourth game, if it wasn’t Chen Meng’s serve wheel and Chen Meng’s serve, but Sun Yingsha reversed the game, the result of the following game would be hard to say.
Before the game, when analyzing the match between Chen Meng and Sun Yingsha, I said: Chen Meng was in a particularly good state this time, and Sun Yingsha experienced high-intensity events in a row, and her state declined, which may add a little suspense to this semi-final.
For players with equal strength, the recent competitive state of the players is very important, and the players’ improvisation has a great influence on the results of the game.
Fan Zhendong’s 3-2 reversal of Lin Shidong in the men’s singles quarter-final of WTT Xinxiang Championship last week is a typical example. Lin Shidong got the match point 10-8 in the fourth game, but Fan Zhendong tied the score. Finally, Fan Zhendong reversed 13-11 to win the fourth game and was tied with a big score. Fan Zhendong won the deciding game 11-8. Moreover, Fan Zhendong finally won the men’s singles championship in Xinxiang Championship.
A game, one or two key points, may lead to a world of difference.
In table tennis, besides paying attention to who wins and who loses, it is better to look at the score of each game.
I’m interested in going to see the players’ respective service wheels, return balls and so on. If you are still interested, then learn about various services, techniques and tactics, table tennis equipment and so on.
So, don’t stop at the level of "0-4 lost". Players are improving, should old fans keep pace with the times?
As for some "extreme fans", they think that the players they like can only win but not lose. Losing is abnormal and fishy. Don’t apply the "rice circle" to competitive sports. Isn’t it good to chase the stars in the entertainment circle?
In the past few years, artificial intelligence (AI) has made great progress, and AI’s new products include AI image generator. This is a tool that can convert input statements into images. There are many AI tools for text-to-image conversion, but the most prominent ones are DALL-E 2, Stable Diffusion and Midjourney.
DALL-E 2 is developed by OpenAI and the project of chatgpt is complementary. It generates images through a paragraph of text description. Its GPT-3 converter model trained with more than 10 billion parameters can interpret natural language input and generate corresponding images.
DALL-E 2 mainly consists of two parts-converting user input into a representation of an image (called Prior), and then converting this representation into an actual photo (called Decoder).
The text and images used in it are embedded in another network called CLIP (Contrast Language-Image Pre-training), which is also developed by OpenAI. CLIP is a neural network that returns the best title for the input image. What it does is the opposite of what DALL-E 2 does-it converts images into text, while DALL-E 2 converts text into images. The purpose of introducing CLIP is to learn the connection between visual and text representation of objects.
DALL-E 2′ s job is to train two models. The first one is Prior, which accepts text labels and creates CLIP image embedding. The second is Decoder, which accepts CLIP image embedding and generates images. After the model training is completed, the reasoning process is as follows:
The input text is converted into CLIP text embedding using neural network.
Use Principal Component Analysis to reduce the dimension of text embedding.
Create an image embedding using text embedding.
After entering the Decoder step, the diffusion model is used to embed the image into an image.
The image is enlarged from 64×64 to 256×256, and finally enlarged to 1024×1024 by using convolutional neural network.
Stable Diffusion is a text-to-image model, which uses CLIP ViT-L/14 text encoder and can adjust the model through text prompts. It separates the imaging process into a "diffusion" process at runtime-starting from the noisy situation, gradually improving the image until there is no noise at all, and gradually approaching the provided text description.
Stable Diffusion is based on Latent Diffusion Model(LDM), which is a top-notch text-to-image synthesis technology. Before understanding the working principle of LDM, let’s look at what is diffusion model and why we need LDM.
Diffusion Models, DM) is a generation model based on Transformer, which samples a piece of data (such as an image) and gradually increases the noise over time until the data cannot be recognized. This model tries to return the image to its original form, and in the process, it learns how to generate pictures or other data.
The problem of DM is that powerful DM often consumes a lot of GPU resources, and the cost of reasoning is quite high due to Sequential Evaluations. In order to train DM on limited computing resources without affecting its quality and flexibility, Stable Diffusion applies DM to powerful Pre-trained Autoencoders.
On this premise, the diffusion model is trained, which makes it possible to achieve an optimal balance between reducing complexity and preserving data details, and significantly improves the visual reality. The cross attention layer is introduced into the model structure, which makes the diffusion model a powerful and flexible generator and realizes the high-resolution image generation based on convolution.
Midjourney is also a tool driven by artificial intelligence, which can generate images according to the user’s prompts. MidJourney is good at adapting to the actual artistic style and creating images with any combination of effects that users want. It is good at environmental effects, especially fantasy and science fiction scenes, which look like the artistic effects of games.
DALL-E 2 uses millions of image data for training, and its output results are more mature, which is very suitable for enterprises to use. When there are more than two characters, the image generated by DALL-E 2 is much better than that generated by Midjourney or Stable Diffusion.
Midjourney is a tool famous for its artistic style. Midjourney uses its Discord robot to send and receive requests for AI servers, and almost everything happens on Discord. The resulting image rarely looks like a photo, it seems to be more like a painting.
Stable Diffusion is an open source model that everyone can use. It has a good understanding of contemporary art images and can produce works of art full of details. However, it needs to explain the complex prompt. Stable Diffusion is more suitable for generating complex and creative illustrations. However, there are some shortcomings in creating general images.