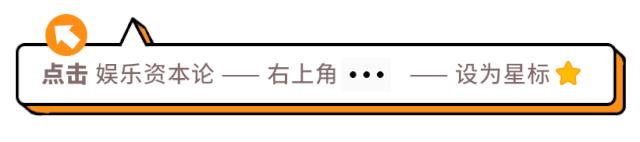
Author/Yali Wang Banxian Editor/Guo Ji ‘an
This year’s National Day seems like the Spring Festival.
Not only the high-speed traffic jams and the crowds of scenic spots make people feel this way, but Together, which ended on the last day of the holiday, also brings people back to the scene of the epidemic raging during the Spring Festival in the form of images.
From "The Turning Point of Life" to "Wuhan People", ten units show the familiar or unfamiliar anti-epidemic stories of the public, and also break all kinds of doubts about "Together".
Are we going to sing a hymn again?
In the broadcast story, "Together" did not avoid the shortage of medical materials and the lack of timely disclosure of government information during the outbreak. The unit of "Turning Point of Life" spoke the line that "it will be too late to wait for the release of CDC" with the mouth of the protagonist Zhang Hanqing, and there were fragments of nurses crying about "rotten masks" and "garbage bags" in "Ferry Man".
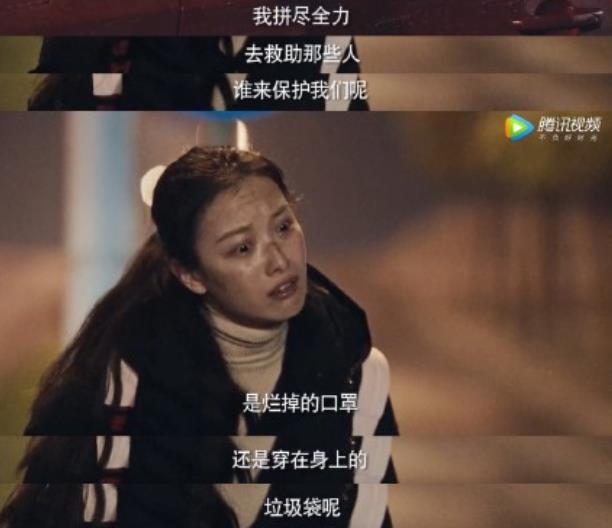
Why not make a documentary and restore it?
In fact, almost all the protagonists in the ten units of Together have real prototypes. The prototype of Zhang Hanqing played by Zhang Jiayi is Zhang Dingyu, the director of gradual freezing disease, and the prototype of Li Jianhui played by Zhu Yawen is Zhong Ming, the first aid doctor in Hubei. The bridge section in the play, which was questioned by some viewers because of riding a bicycle back to Wuhan, is actually the true story of the prototype doctor Gan Ruyi.
Are you going to tear up again?
Different from what the audience thought, "Together" is extremely restrained, even diluting the sad mood to some extent. When Zhang Hanqing, the old dean, learned that his friend Liu Yiming, who was also the dean of the hospital, was infected and died, the camera only gave a short silence and a distant view. When the takeaway Gu Yong decided to go to the hospital to deliver food, there was only one sentence, "Isn’t it necessary to deliver food now?"
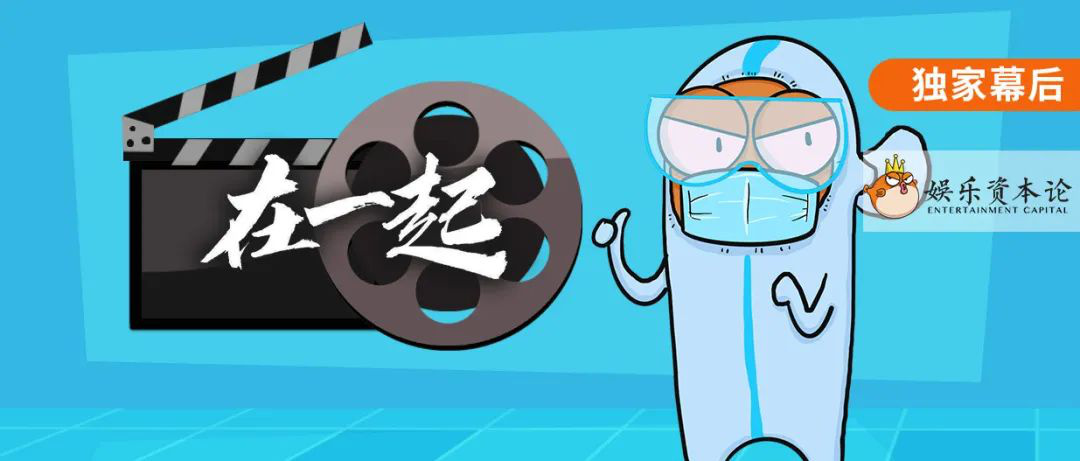
In order to restore the true, restrained and unavoidable birth process of Together, Entertainment Capital exclusively interviewed Sun Hao, the producer of this drama, and Echo Gao, the screenwriter of The Ferry Man, Liu Liu, the screenwriter of Fangcang and Yang Yang, the director of Wuhan Man, to connect the twists and turns behind the filming.
Preparation:
Shoot unit dramas, write little people, and don’t just sing hymns.
On February 16th, just three days after taking office, Gao Changli, the current director of the TV drama department of the State Administration of Radio and Television, came up with the idea of making a drama to record the epidemic. He found Gao Yunfei, then director of Shanghai Radio and Television (now deputy director of the Propaganda Department of the Shanghai Municipal Committee of the Communist Party of China), and prepared to start the later Together. When Gao Yunfei gave a speech at the Shanghai TV Festival Forum, he specifically mentioned "the urgency of creation" and hoped that the series could be broadcast in October this year. It was Yaoke Media, which had made great achievements in realistic dramas, that took over the tasks from SARFT and Shanghai Radio and Television Station.
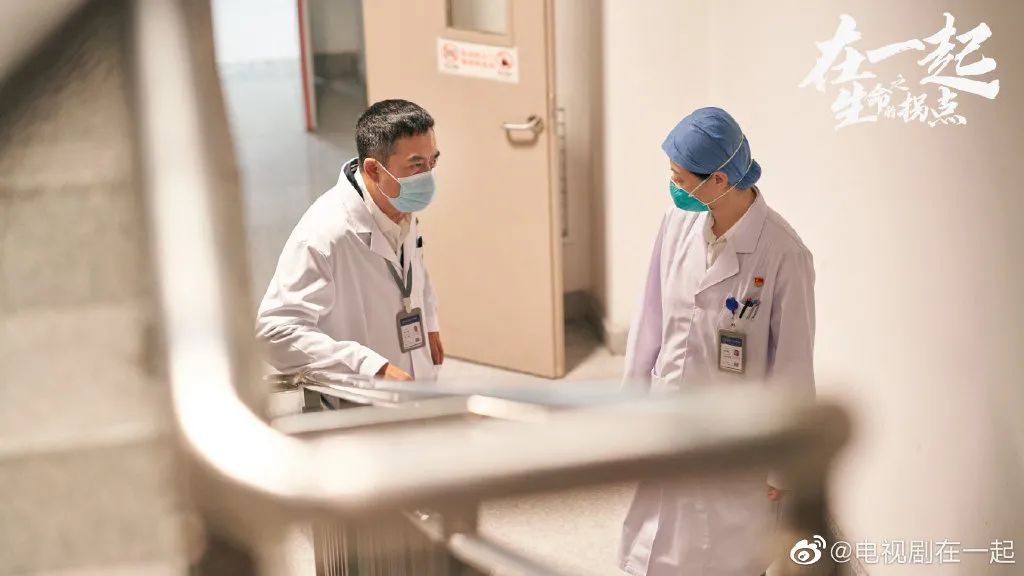
However, according to the production process of traditional long drama series, even a 20-episode script needs several months of polishing, plus the shooting cycle, time is simply not enough. So the producer thought of My People,My Country, a tribute film composed of seven short films. "We thought it was quite suitable for this task, so we put forward the idea of unit drama to the General Administration and Shanghai Radio and Television Station. Each unit has two episodes, focusing on a special group and telling a relatively independent story." After getting the approval of the leader, the production team began to plan in detail. Generally speaking, the creation principle of Together follows three points: true restoration, writing little people, and not blindly singing hymns. Gao Changli, director of the TV drama department, is a documentary, and "truthfulness" is his basic requirement for TV dramas. Chen Yuren, the current vice president of Shanghai Culture, Radio, Film and Television Group Co., Ltd., also said in an interview that he was in charge of documentary work in SMG and could understand the concept of "Time Report Drama" proposed by Gao Changli. "Together" takes "truth" as the bottom line from the competent unit to the production team, unifies the documentary style and implements the concept of "Time Report Drama". So on the basis of giving consideration to the coverage of the industry, the film chose the most representative news figures or groups. For example, the first unit "The Turning Point of Life" shows the deeds of local doctors in Wuhan, while the unit "Rescuers" tells the story of the medical team assisting Hubei.
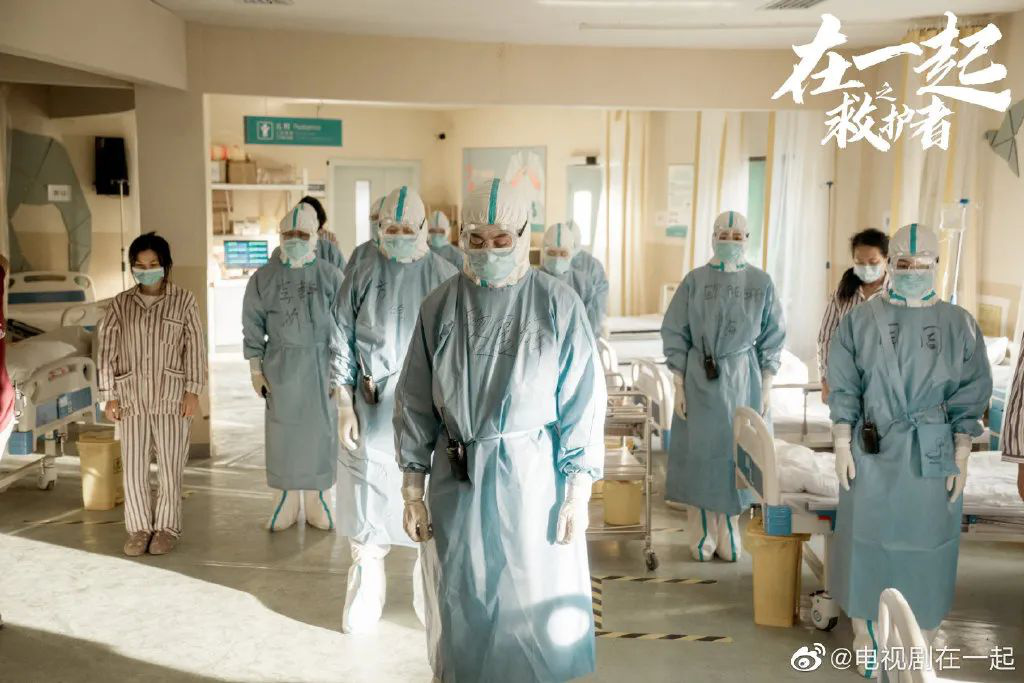
Unit stills of "Rescuers"
At that time, there was a rumor on the Internet that there would be a story about Zhong Nanshan, which was played by Chen Daoming, and the film side quickly refuted it. Sun Hao explained the theory of entertainment capital. "When we communicated with the General Administration and Shanghai Radio and Television Station, the consensus was to write stories about little people. Academician Zhong Nanshan made great contributions, but we chose ordinary people and groups." After the master plan was submitted, SARFT and Shanghai TV Station selected nine stories and returned an opinion, hoping to join the unit of "Wuhan people". Although the stories reported by the film reflect people from all walks of life who went to rescue, the local residents in Wuhan who really bear the greatest pressure in the epidemic need to be seen. Ten stories were settled, and the film began to form a team and invited writers, directors and actors. According to the theory of entertainment capital, when choosing a screenwriter, the film will give priority to the scriptwriters who have cooperated. Screenwriters who have not cooperated with each other will hope to be first-line screenwriters and be good at creating realistic themes.
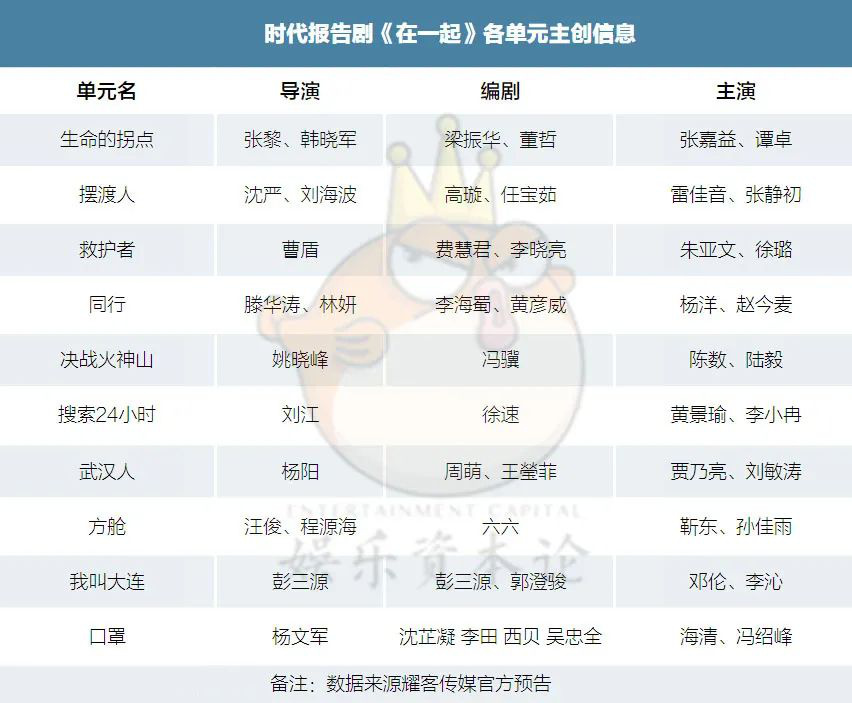
But Together is a task drama, not only the audience will have a lot of speculation, but also the screenwriter will hesitate. "Some screenwriter teachers may worry that this will be too main theme. But we will all emphasize to the screenwriter that this is not just singing hymns. " Sun Hao said. Liu Liu was reluctant to take this project at first. "I was in Singapore at that time, and I had to fly back with my family." And according to the creative principle of June 6th, she never writes stories without personal feelings and observation. In order to invite her to join, the producer promised her to go to Wuhan for field research in person. In contrast, the invitation of Echo Gao, the screenwriter of The Ferry Man, went smoothly. "I have been paying attention to the situation of the epidemic, and I have also paid attention to the group of sellers and couriers in the morning. Even if we didn’t take "Together", the takeaway brother who was driving alone in the city of Wuhan under the epidemic and maintained the life of this city was also a story we wanted to write. " Echo Gao said. The two sides hit it off. As a director, Shen Yan was moved by the little perspective and real texture of The Ferry Man and wanted to join. However, when he received the invitation, another play directed by him was about to start in Shanghai. If he went to Wuhan to shoot, he had to be isolated for 14 days when he returned to Shanghai, which would inevitably delay the start of this play. Just as he was worried about the schedule, the filming location of "The Ferry Man" was set in Shanghai under the coordination of all parties, and director Shen Yan joined smoothly.
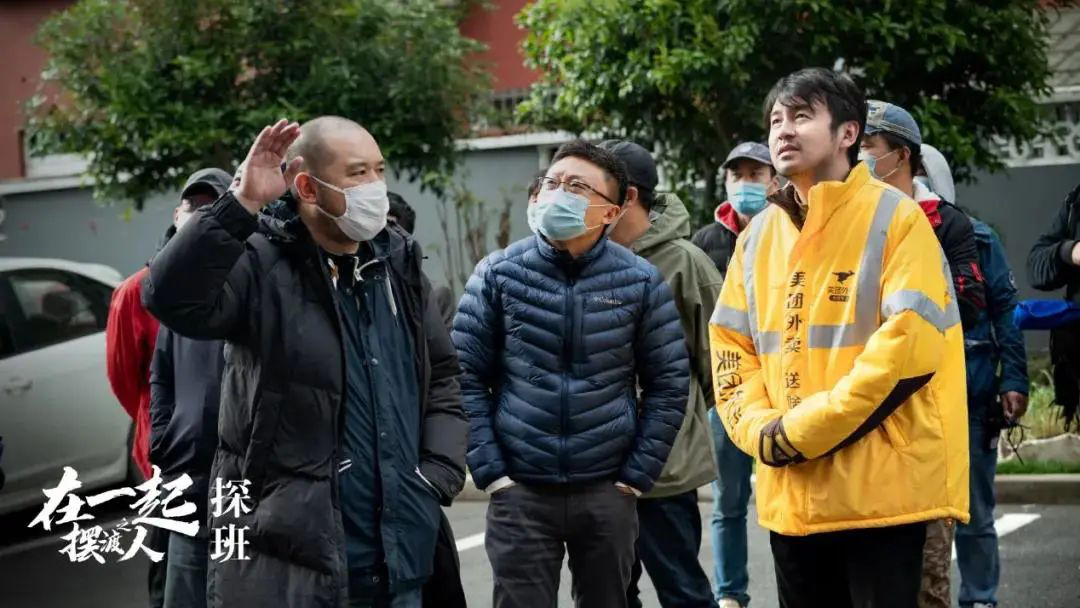
So "The Ferry Man" became the earliest finalized, filmed and completed crew, and the film side also set up the creative team of 10 stories. Finally, in order to ensure the unity of the stories, ten stories were edited by one editor, and the soundtrack was also produced by one person. The attentive audience may find that Lei Jiayin, the protagonist of another unit, The Ferry Man, appeared in the unit "The Turning Point of Life". This is also the intention of the creative team. "We have linked the plots of some units and hope to have a more overall presentation." From an idea of Gao Changli to the upcoming production of a whole drama, Together is indeed an exaggeration to call it "a great gathering of China film and television circles". The overall operation is not easy, and the creation and shooting process of individual projects also have their own difficulties.

Creation:
Online meetings, real records, and no deliberate pursuit of conflict.
The problem faced by screenwriter Liu Liu is that he is a practical school and must experience it personally before he can write. However, coming to Wuhan in March when the epidemic situation has not been completely controlled is bound to face certain risks and many inconveniences. At first, Liu Liu just planned to look at it before deciding whether to write or not. However, after staying in Wuhan for a few days, Liu Liu’s feelings are getting deeper and deeper. Wearing suffocating protective clothing, nurses dragging dozens of kilograms of oxygen bottles, doctors lifting weights in dignified atmosphere … As time went on, her feelings became deeper and deeper. Twenty days later, she left Wuhan and began to write the first edition of the script.
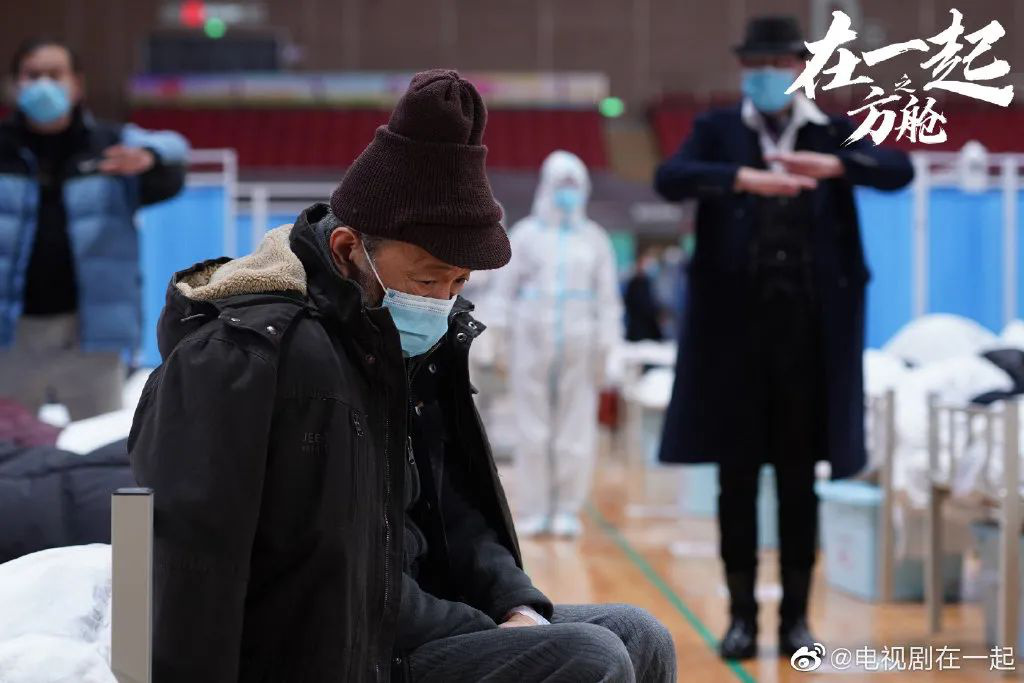
Holding a huge amount of material, which ones are suitable for the script? The standard of "June 6th" is that the material obtained through interviews can be precipitated and recalled repeatedly. "There is no need to compile, and the real picture will always hover in my mind." It only took her a week to produce two episodes of the script. Echo Gao, a screenwriter, also collected a lot of materials and felt that it was unnecessary to compile them. Everything is true. Weibo, who comes from news reports and front-line delivery staff, is an ordinary drop in life. "Long before receiving this project, many on-the-spot reports about the delivery staff were engraved in her mind. In her opinion, this kind of creation without fabrication is the most consistent with the law of creation. After receiving the creative task of Together, screenwriters Echo Gao and Ren Baoru conducted an in-depth interview with the Wuhan takeaway. During the epidemic, many Wuhan takeout workers insisted on sharing their stories about the city closure on Weibo: some mothers with children at home asked them to buy thermometers, some medical staff who worked overtime in the hospital asked the takeout workers to deliver food, and more ordinary people who were grounded at home asked them to buy vegetables. In the end, Echo Gao "pinched" these delivery couriers into one person-Gu Yong, a takeaway brother played by Lei Jiayin in The Ferry Man. "He is the most common takeaway brother, who can only bring little help to others, such as sending a meal, bringing a mask and a bottle of alcohol, and that’s all. They are the most ordinary heroes in this city. " Echo Gao said.
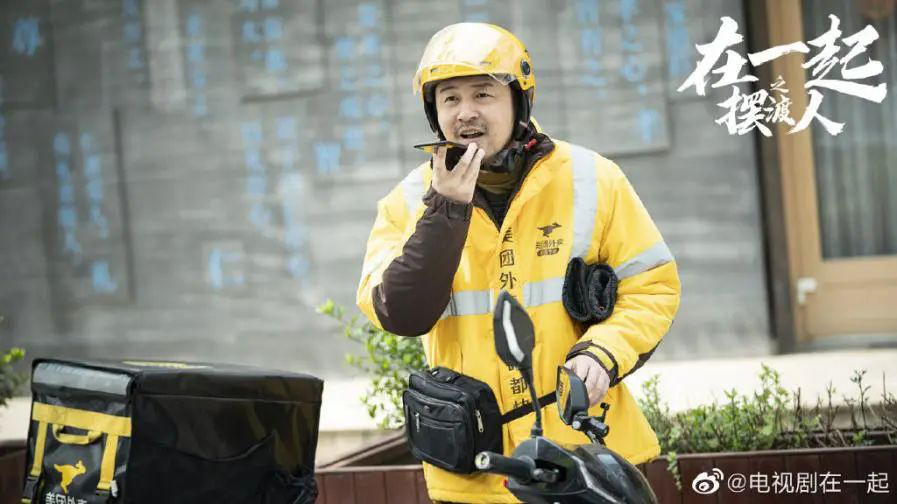
Unit stills of The Ferry Man
Traditional TV drama creation pursues dramatic conflict, but The Ferry Man also selects many subtle and moving points of life. These details, which do not constitute the ultimate dramatic conflict, also have the power to touch people’s hearts. Echo Gao still remembers a real detail: a delivery clerk went to deliver the food. On the empty Wuhan street, he saw an old couple helping each other across the street. The old couple were very surprised to see the takeaway brother. They asked him from a distance: "Is there any takeaway now?" The little brother replied: "Yes! Always! " After receiving a positive reply, the two old people were very happy, as if their lives were dependent. Echo Gao wrote this real life detail into The Ferry Man.
Yang Yang is the most close relationship between the creative team and Wuhan. She was supposed to come to Wuhan in January this year to collect materials for another play. But because of the epidemic, she finally diverted to other cities and interviewed other people related to the story of the play. In the blink of an eye, in late February, the producer called and asked her to join the project Together. Because the epidemic situation in Wuhan is still serious, the unit of "Wuhan People" was finally selected to shoot in Tianjin. Yang Yang and screenwriters Zhou Meng and Wang Yingfei can only interview community workers in Wuhan online.
During the preparatory period, almost every creative meeting was held online. The relevant leaders of TV drama department, SMG, Dragon TV, and all the creators’ teams, totaling 30 to 40 people, reported the progress one by one in the afternoon, and got suggestions on the scale control and the revision of the story content. It was not until May that the whole creative team had the first offline class visit and exchange. In order to create a sense of reality, the crew of "Wuhan People" did not use a shed or a tripod, and used a hand-held lens throughout. "Have that kind of unstable breathing." Yang Yang said. Actor Liu Mintao recorded the Wuhan dialect and studied it every day. Buying food, walking the dog, buying medicine … It is these trivial things that make up Wuhan People.
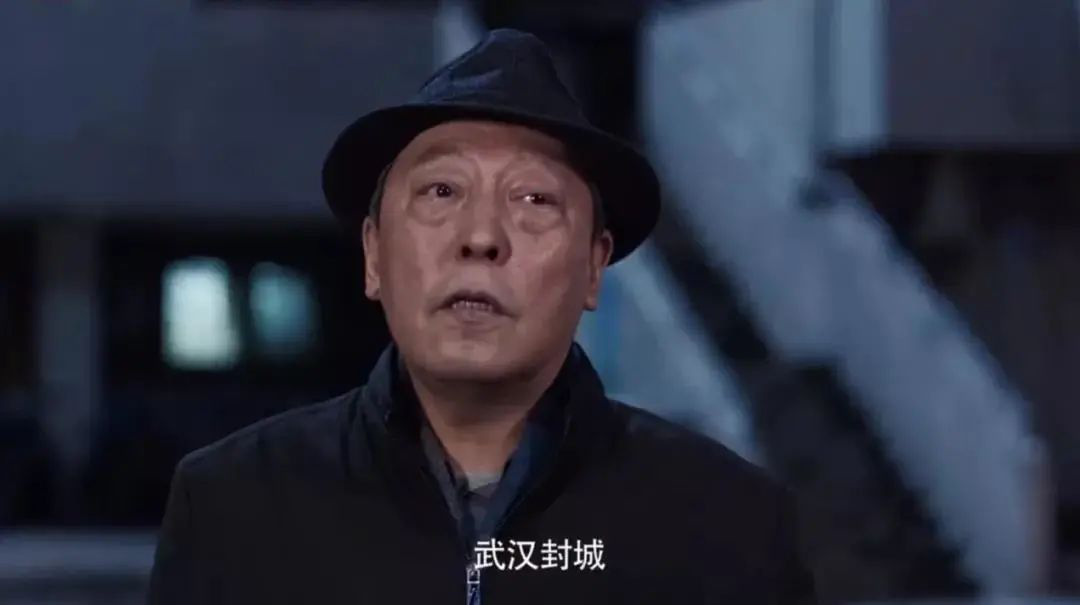
Screenshot of unit preview of Wuhan People
Shooting:
There are many co-shooting departments, tight schedule of actors and off-season shooting.
Among the 10 units, except for the unit "The Final Battle of Vulcan Mountain", the other 9 units have entered the later stage. The reason for the late start-up is that the content of "Battle of Vulcan Mountain" involves troops, and not only the script needs the cooperation of troops, but also the coordination of troops when shooting. Sun Hao, the producer of Together, told Entertainment Capital that there are three main difficulties in the filming process: first, there are many departments that need coordination; second, it is difficult to coordinate the schedule of actors; and third, anti-season filming. Take coordinating screenwriter Liu Liu to go to Wuhan on March 8 as an example. In order to ensure Liuliu can go to Wuhan, the production team coordinated National Health Commission, Shanghai Propaganda Department, Hubei Radio and Television Bureau and other departments. Finally, Shanghai Anti-epidemic Command and Wuhan Anti-epidemic Command joined in, and the joint efforts of many parties finally contributed to the trip to Wuhan in June.
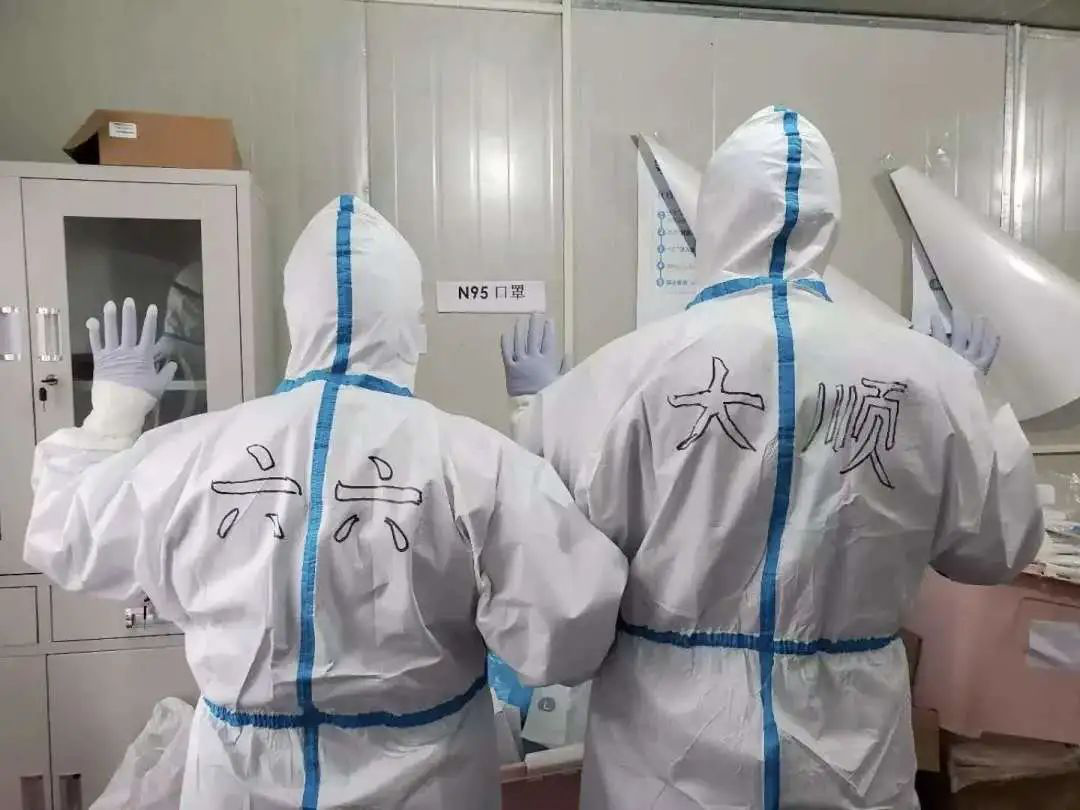
Image from WeChat official account Yaoke Media
And the coordination of medical equipment. Ten crews are scattered all over the country, and some of them shoot real scenes, such as the hospital in The Turning Point of Life, and some can only set the scene because of the shooting conditions, such as The Rescuers, which started in Xiangshan. Not only does the ward need to be built, but also manufacturers of medical devices need to be contacted through National Health Commission and transported to Xiangshan from all over the country. Song Jiong-ming, director of Shanghai Radio and Television Station, mentioned in a speech: "In order to strive for the ultimate scene restoration, the ICU wards in the" Rescuers "unit were all built in Xiangshan, Zhejiang Province. We also invited seven first-line medical staff to participate in the guidance and participation at the scene, and put limited costs on production."
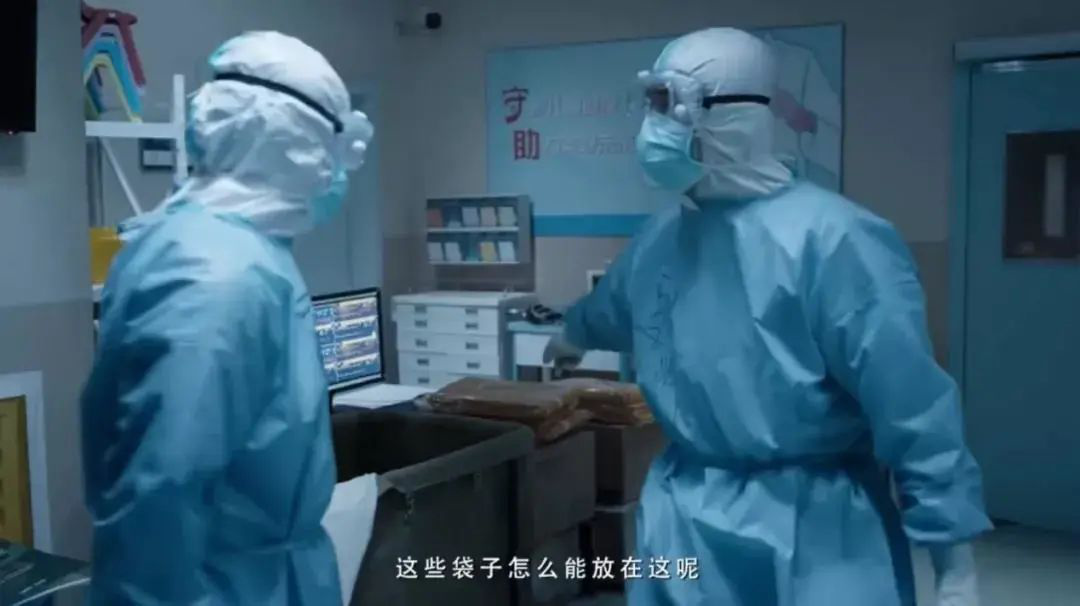
Screenshot of unit preview of "Rescuers"
As for the schedule of actors, The Ferry Man started in early April, when most of the actors had not returned to work, and Lei Jiayin happened to be filming Extreme Challenge in Shanghai. When he received the invitation, he joined the crew of Together without hesitation. This time, some coincidental casting has created a very good effect. The delivery clerk played by Lei Jiayin has a messy hair, which has brought a strong sense of substitution to the audience both in image and performance.
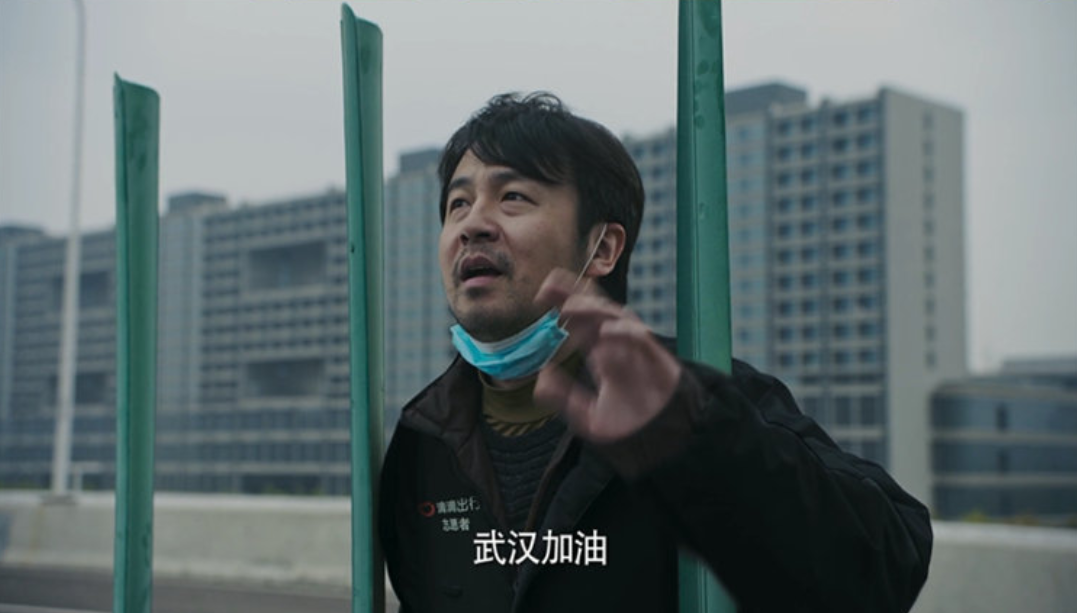
However, it is also difficult to start early. At that time, the epidemic was still serious. Even in Shanghai, where the epidemic was well controlled, the choice of scenes was restricted everywhere. By June and July, it will be difficult to knock the actors again when the big plays are started one after another. Everyone can only work overtime. Take Wuhan Man directed by Yang Yang as an example. The crew started shooting in early July, and the longest time was 22 hours in continuous shooting. "It was the scene of moving vegetables in the rain in the middle of the night. Jerry almost flashed his waist when moving vegetables. Liu Mintao was so thin that he had to take such a big bag of potatoes." The only thing that is fortunate is that the epidemic situation in Xinfadi, Beijing broke out again in June. Although all the scenes that the crew found in Tianjin in advance were shot, the actors were just not in Beijing and were not isolated. As a result, the crew temporarily re-searched the scenery in Tianjin and finally found a community that did not exclude Beijingers from filming. Off-season shooting is a problem that most crews will encounter. It is normal to wear down jackets in the hot sun, and the protective clothing is hot and airtight, but in order to save time, many actors don’t take it off when they rest. In order to cover up the scorching sun in July, the crew of Wuhan People changed most of the exterior scenes into night scenes. In order to restore the rainy weather in Wuhan in January and February, sprinklers should sprinkle water at any time. In some places, sprinklers could not get in, so the crew simply splashed water on the washbasin.
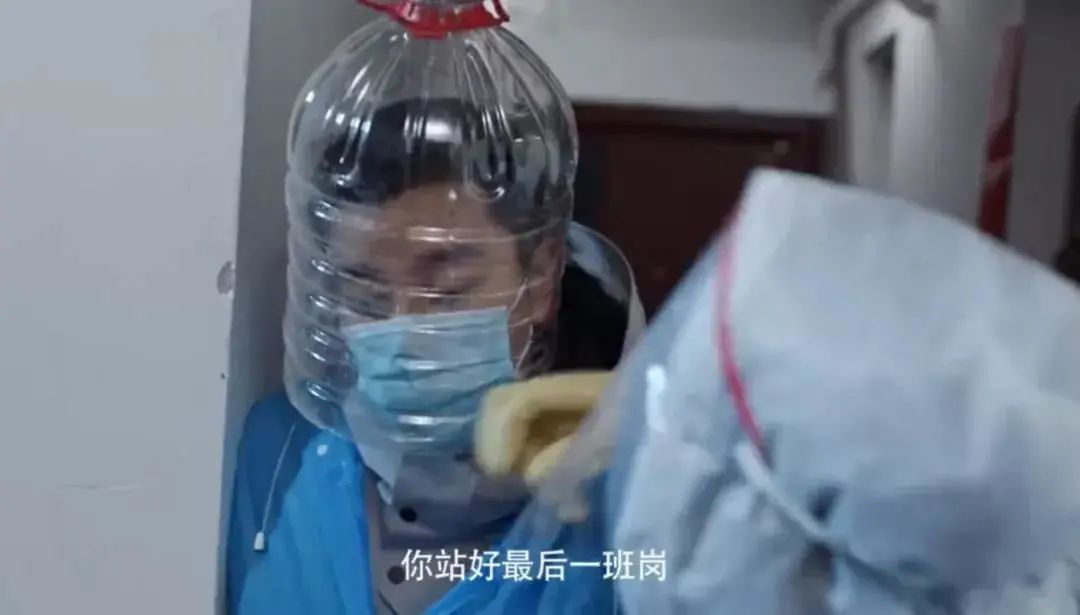
Screenshot of unit preview of Wuhan People
Sometimes, the crew will receive "complaints" from community residents. July is the key period for students to prepare for the college entrance examination this year, and the crew often shoot big night plays, which will inevitably make a noise. In order to reduce the decibel, the crew changed the "action" shouted during shooting to gestures, trying to identify each other’s body language in the dark.
Less than a month after the filming of Wuhan People, Yang Yang really went to Wuhan to prepare another play. Taking a taxi from the airport to the hotel, she passed the Yangtze River Bridge and the Yellow Crane Tower … and passed many Wuhan landmarks she mentioned in Wuhan People. She looked at these scenes seriously, as if she had been here many times. When I came to the hotel where I stayed and lay in bed, Yang Yang was deeply moved. She didn’t come to Wuhan in January because of the epidemic, but she met many experts and scholars who had contacted Wuhan people. During the Spring Festival, Yang Yang’s mother suddenly coughed and had a fever. She quickly took her mother to the Union Medical College Hospital, but she didn’t queue for four hours. "At that time, I was particularly worried that I was infected with her." Until now, when she was really lying on the bed in Wuhan Hotel, she suddenly felt a specious feeling. The experience of the past six months flashed through her mind like a movie. The story of Wuhan people is also the story of me and many people, and what they have experienced is also the experience of many of us. "Yang Yang said. This may also be the reason why everyone is scrambling to participate in the "Together" project. Not because of the task, nor to sing a hymn, but to leave a collective memory of China people.
Leave a message for discussion
What is the most touching part for you?
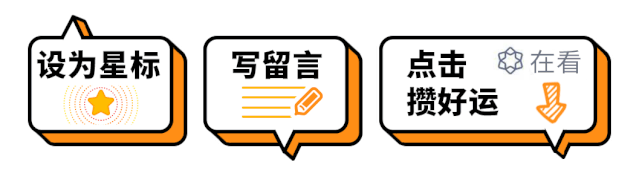